Crypto x AI: The intersection of two transformative technologies
Introduction
Artificial intelligence has a rich history that dates back to the mid-20th century. What began as theoretical models and rule-based systems has evolved into today’s landscape of neural networks, generative AI, and autonomous decision-making. From chatbots to self-driving cars, AI systems are solving increasingly complex problems, demonstrating capabilities that were once confined to science fiction. Yet, with this rapid progress comes a host of limitations and dangers. AI models are often opaque, leading to concerns about bias and accountability. Moreover, their reliance on vast amounts of data raises ethical questions about privacy and data ownership. Recent headlines have also highlighted risks, such as the misuse of generative AI to create misinformation or deepfakes.
These challenges prompt an important question: How can we build systems that are not only intelligent but also transparent, secure, and equitable? This is where the intersection of AI and blockchain technology comes into play. Blockchain—the decentralized ledger system that underpins cryptocurrencies—offers unique features like transparency, immutability, and decentralized governance. By integrating blockchain with AI, we can address some of AI’s most pressing limitations, creating systems that are both more trustworthy and resilient.
In the sections that follow, we’ll delve deeper into the current state of centralized & decentralized AI, outline its challenges, and explore how blockchain technology can serve as a complementary force. Finally, we will also explore the disadvantages of decentralized AI compared to its centralized counterpart.
Comparing the current AI stack and the decentralized AI stack
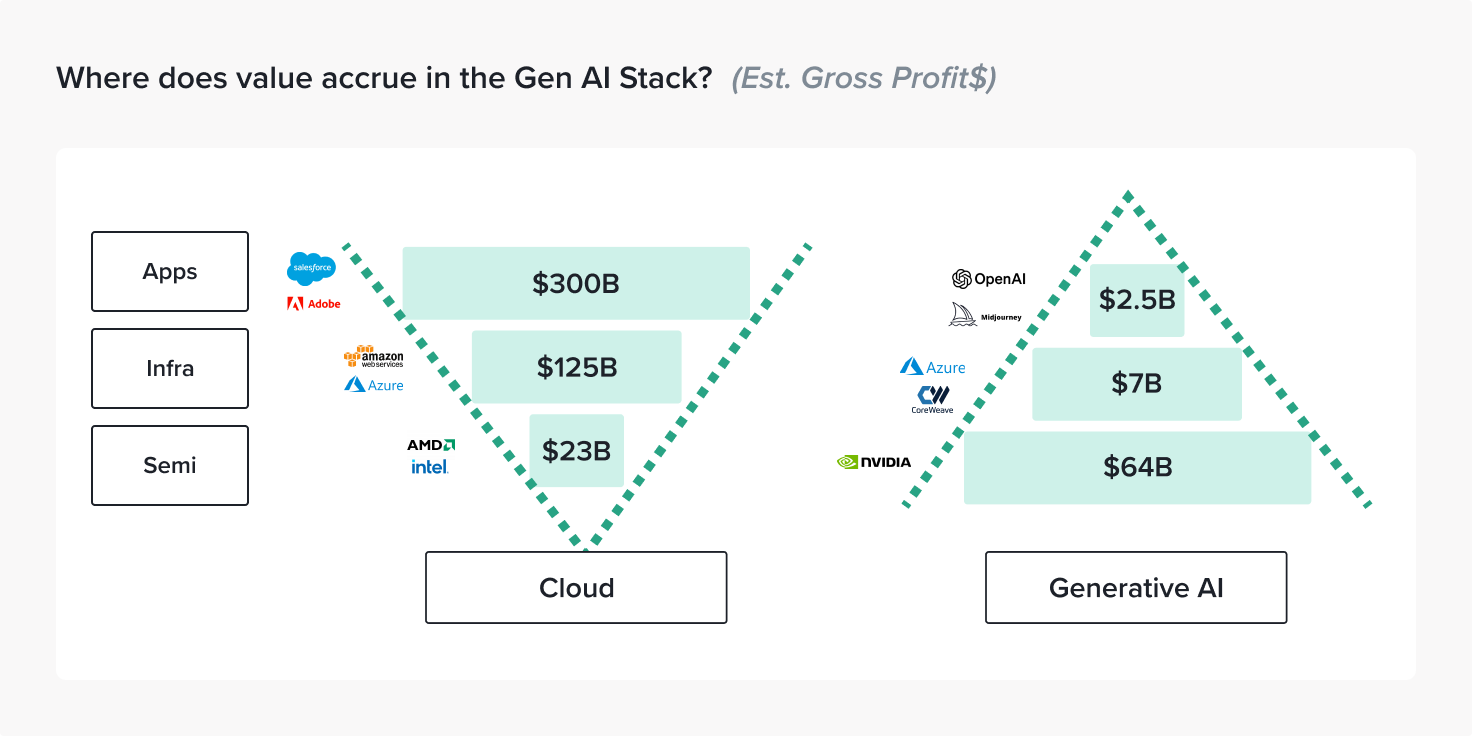
Source: Altimeter
Layer 1: The Hardware Foundation
The Current AI Stack begins with the semiconductor layer, dominated by Nvidia. With a staggering 90% market share, according to Yahoo Finance (source), in data center GPUs, according to Yahoo Finance, Nvidia's hardware forms the backbone of generative AI by enabling the computation-intensive tasks required for training and deploying complex models. Although it plays a leading role, this centralization comes with challenges, including supply chain vulnerabilities, higher costs, and the risk of monopolistic tendencies. Depending on a single provider can create constraints that may limit competition and innovation.
In contrast, the Decentralized AI Stack also starts with a hardware layer but emphasizes diversity and trust. This layer incorporates tokenized GPU solutions and decentralized hardware to ensure verifiability and accountability. By distributing the infrastructure, it mitigates centralization risks, but it also faces challenges such as ensuring interoperability and achieving the same level of performance efficiency as centralized counterparts.
Layer 2: Computational and Storage Infrastructure
The Current AI Stack employs cloud computing giants like AWS, Microsoft Azure, and Google Cloud as the infrastructure layer. These platforms provide scalable environments for training and deploying AI models, with specialized inference providers like CoreWeave offering tailored solutions. However, this scalability comes at a cost: high operational expenses and potential risks around data privacy and centralized control. Moreover, this layer’s heavy reliance on centralized services can expose organizations to systemic risks like outages or regulatory interventions.
The Decentralized AI Stack replaces centralized cloud services with a physical compute- and storage layer built on decentralized infrastructure. By leveraging distributed networks, it offers enhanced robustness, reduced dependency on single points of failure, and increased data sovereignty. However, it must contend with latency issues, resource allocation inefficiencies, and the complexities of managing decentralized governance models for infrastructure provisioning.
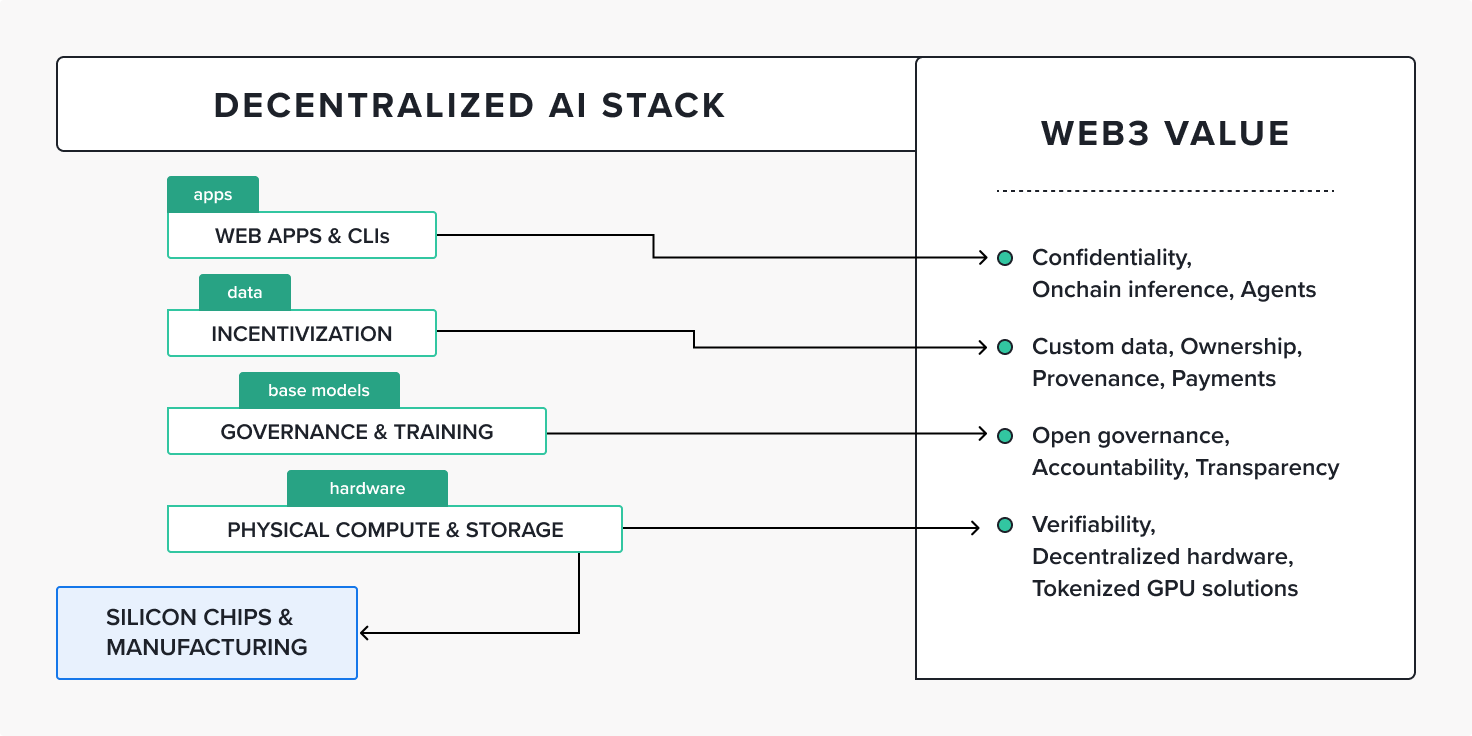
Source: Oasis Protocol
Layer 3: Governance, Training, and Incentivization
For the Current AI Stack, governance and training are implicit within the ecosystems of large enterprises and research institutions. While this provides centralized control and efficiency, it also raises concerns about transparency, bias, and accountability in model development. Training datasets can often lack diversity or provenance tracking, which may lead to models with inherent biases or vulnerabilities.
The Decentralized AI Stack integrates governance and training within a distinct layer that prioritizes transparency and community participation. Open governance frameworks allow stakeholders to contribute to and oversee decision-making processes. Additionally, provenance tracking ensures datasets are ethically sourced and contributors are rewarded through tokenized incentives. However, these systems can be slower to implement, and balancing inclusivity with efficiency remains a significant challenge.
Layer 4: The Application Layer
At the top of the Current AI Stack are generative AI applications such as OpenAI’s GPT models, Midjourney’s image generation platform, and enterprise AI tools. These applications capture public imagination and drive business value. However, their reliance on proprietary systems can limit transparency, restrict user customization, and create dependencies on specific vendors.
The Decentralized AI Stack introduces an application layer that prioritizes user sovereignty and security. This layer supports web applications and command-line interfaces that integrate features like confidentiality, on-chain inference, and autonomous agents. While this design fosters user-centricity and reduces reliance on centralized systems, it also requires significant advancements in usability and performance to match the seamless experience of centralized applications.
Crypto x AI Ecosystem
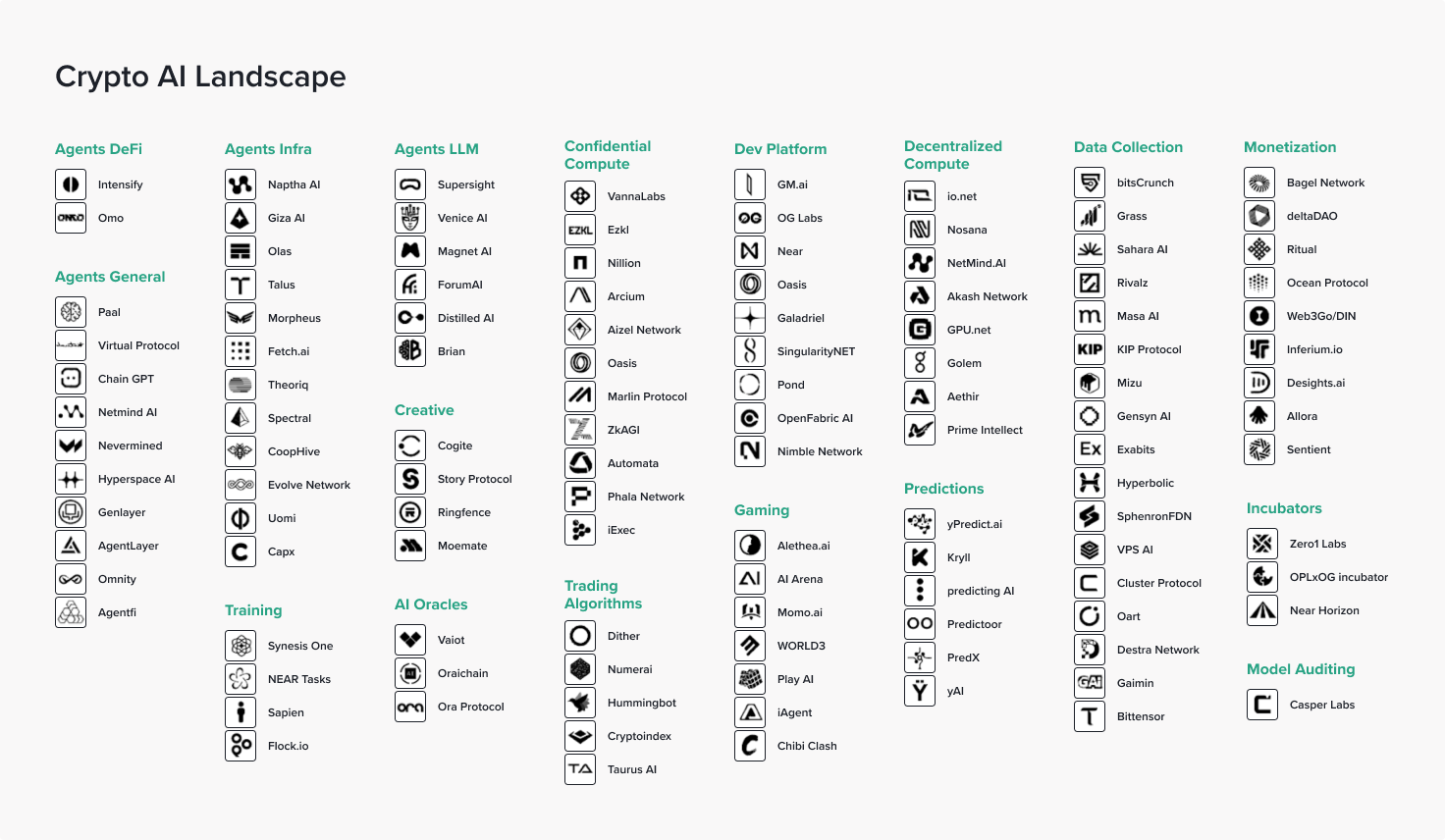
Source: Oasis Protocol
Agents and Autonomous Systems
Autonomous AI agents are intelligent systems capable of performing tasks independently without human intervention. These agents can perceive their environment, process data, and make complex decisions with a high level of adaptability (Binance Research). In the context of blockchain and decentralized systems, AI agents are revolutionizing various aspects of the crypto ecosystem.
Autonomous trading and investment agents are at the forefront of this innovation. These agents can analyze vast amounts of data, recognize patterns, and execute trades in real-time, optimizing portfolio management and market analysis (Forbes). For instance, AI agents in crypto can interact with smart contracts and blockchain data to autonomously perform tasks such as token swaps and liquidity provision, operating continuously in the 24/7 crypto markets. Some examples of AI agent frameworks are Virtuals.io or the latest Eliza OS by ai16 z, which has been getting a lot of attention lately.
Decentralized decision-making and governance agents are transforming how blockchain communities function. Through on-chain voting mechanisms and Decentralized Autonomous Organizations (DAOs), token holders can directly participate in shaping a project's direction. AI agents can facilitate this process by analyzing proposals, assessing their potential impact, and even generating governance suggestions based on community sentiment and project goals (Medium).
Security and monitoring agents play a crucial role in maintaining the integrity of blockchain networks. These agents can continuously audit blockchain applications for vulnerabilities, detect fraudulent activities, and enhance overall system security (D4 Insight). By leveraging machine learning and large language models, these agents can adapt to new threats and provide real-time protection against evolving security risks.
Decentralized Compute
Decentralized hardware marketplaces are another prime example which have emerged as a solution for AI developers and researchers, offering access to high-performance computing resources without the need for substantial upfront investments. These platforms enable individuals and organizations to rent powerful GPUs and other specialized hardware for training AI models, democratizing access to cutting-edge technology.
One example in this space is Prime Intellect, a German project that is building infrastructure for decentralized AI development at scale. Prime Intellect aggregates global compute resources, allowing researchers to collaboratively train open AI models and share in their ownership and benefits. Their platform, currently in beta, offers cost-effective, scalable on-demand compute by aggregating supply from various GPU providers (Prime Intellect).
Another significant player is Aethir, a decentralized cloud computing platform that aggregates GPU resources from enterprises, data centers, miners, and vendors. Designed with a specialized GPU framework, Aethir creates a unified network that increases cluster size and improves infrastructure reliability. The platform labels its mission as closing the “AI Wealth Gap” (Messari).
Data and Training
The ecosystem is tackling the challenge of data scarcity for AI model training. Projects like Masa AI and Grass are working on data monetization and decentralized web scraping. For training, initiatives such as Synesis One are enabli ng incentivized and verifiable training mechanisms on-chain.
Developer Platforms and Gaming
Platforms like 0G Labs and NEAR are actively attracting developers by offering robust tools and infrastructure to streamline AI stack integration. These platforms empower developers to build scalable and decentralized AI applications with minimal friction, enabling faster deployment and experimentation. In the gaming sector, projects such as Alethea.ai and AI Arena are pushing boundaries by utilizing AI to create more immersive and interactive gaming experiences. For instance, Alethea.ai focuses on AI-generated characters that can interact dynamically with players, while AI Arena combines gaming with education, teaching users about AI concepts through engaging gameplay.
Monetization and Predictions
The crypto x AI ecosystem is rapidly innovating in monetization and predictive analytics. Projects like deltaDAO and Ocean Protocol have pioneered the creation of decentralized marketplaces for AI models and data, allowing individuals and organizations to monetize their contributions effectively. These platforms ensure fair compensation for data providers and facilitate the sharing of high-quality datasets, fueling AI advancements. In the realm of predictions, platforms such as yPredict.ai and Predictoor leverage AI for highly accurate market forecasts and trading strategies. By analyzing vast datasets in real time, these tools empower traders and investors to make informed decisions, showcasing the practical benefits of AI within decentralized systems.
DeAGI
Artificial General Intelligence (AGI) represents a theoretical form of AI capable of performing any intellectual task that a human can do. Unlike narrow AI systems designed for specific tasks, AGI aims to replicate human-level intelligence across a wide range of cognitive abilities. This concept has long fascinated researchers and could revolutionize industries by solving complex problems and adapting to new situations without specific programming (IMD).
Blockchain technology offers a promising avenue for AGI development. By providing a decentralized, transparent, and secure platform, blockchain enables researchers to share data, code, and resources more effectively (Hackernoon). This collaborative approach could accelerate AGI research by pooling diverse datasets and computational power, while ensuring data integrity and privacy (Singularity NET).
The future intersection of AI and blockchain holds immense potential. Decentralized AGI systems could leverage blockchain's governance capabilities to embed democratic decision-making into their development and deployment. This could address ethical concerns by ensuring AGI remains aligned with human values and benefits society as a whole. As we move towards 2025, the synergy between AGI and blockchain is expected to drive innovation across various sectors, from personalized medicine to financial risk management.
The Downsides
While the intersection of AI and blockchain holds promise, it's crucial to consider potential risks and challenges. One significant concern is data privacy and security. As AI systems require vast amounts of data, integrating them with blockchain could expose sensitive information to all network participants, potentially violating privacy regulations.
Scalability remains a major hurdle. Running AI models on blockchain networks requires significant resources, making it generally more costly than centralized solutions. These higher costs, combined with slower transaction speeds, can impede scalability and real-time processing, which are essential for many AI applications.
Technical complexity poses another challenge. Integrating AI with blockchain requires specialized expertise in both fields, which is often scarce. This skill gap can lead to implementation difficulties and increased vulnerability to security threats (restack).
Furthermore, the regulatory landscape for both AI and blockchain is still evolving. The lack of standardized protocols and regulatory uncertainty creates a complex environment for organizations looking to adopt these technologies (inatba).
Given these challenges, it is crucial to explore the intersection of AI and blockchain with a focus on the unique challenges they present, emphasizing the importance of understanding use cases, assessing risks, and addressing security concerns.
Summary
As we step into 2025, the fusion of AI and blockchain is reshaping our technological landscape. This powerful combination promises to revolutionize industries by merging blockchain's secure, transparent framework with AI's adaptive intelligence. From decentralized decision-making to enhanced data privacy, the possibilities are endless.
While challenges remain, the intersection of AI and blockchain suggests potential for more secure and decentralized technological applications. Rather than a competition, this relationship represents an opportunity to explore how the strengths of both technologies can complement each other.
As this integration evolves, its broader implications and true value are still uncertain. While progress across various sectors is possible, significant hurdles must be addressed before the full potential of this convergence can be realized.
DISCLAIMER
This blog post does not constitute investment advice or a solicitation to purchase any products or other offerings. Any investment decision should be made based on your own research and consultation with professional financial advisors. Past performance is not indicative of future results.